MIXED METHODS AND CROSS-CUTTING APPROACHES
19 Qualitative Comparative Analysis
Valérie Pattyn
Abstract
Qualitative Comparative Analysis (QCA) is a mixed method which translates qualitative data into a numerical format in order to systematically analyse which configurations of factors produce a given outcome. QCA indeed relies on a configurational conception of causality, according to which outcomes derive from combinations of conditions. It is very useful for ex post impact evaluation, more specifically to understand why the same policy may lead to certain changes in some circumstances and not in others.
Keywords: Configurations, combinations of conditions, causal complexity, systematic identification of cross-case patterns, equifinality, conjunctural causation, asymmetrical causality
I. What does this method consist of?
Why is it that the same policy leads to certain changes in some circumstances and not in others? Take, for example, a subsidy programme supporting firms to provide in-company training on leadership skills. Why is it that such training is effective for some employees, and for others not? Or put differently: under what conditions does, or does not, successful ‘training transfer effectiveness’ occur? Qualitative Comparative Analysis (QCA) is a method to answer such a question.
QCA assumes that configurations – i.e. combinations of conditions – are necessary and/or sufficient to achieve a given outcome. Conditions can be conceived as causal variables, determinants, or factors (Rihoux, and Ragin, 2009: xix). An outcome, in an evaluation context, is usually a well-defined intended or unintended policy effect that may be present or absent. In the above example, the outcome is the occurrence or non-occurrence of ‘training transfer effectiveness’.
Different from other case-based methods (See separate chapter on case studies), QCA enables comparison of case-based information systematically, and as such allows for modest generalisation. At the same time, different from statistical methods, it enables us to keep rich contextual information and some complexity. Because of this twofold potential, the method is often portrayed as a bridge builder between qualitative and quantitative methods. The method was originally developed for researchers confronted with an intermediate number of cases (between 10 and 50), but is increasingly also applied in settings with a large number of cases (see Thomann, and Maggetti 2020).
Importantly, QCA is not only an analytical technique, but also comes with a specific approach to causality, labeled as multiple conjunctural causation, which is very compatible with assumptions underpinning realist evaluation (see separate chapter on realist evaluation). In particular, this approach entails that:
-
Policy effects are often the result of combinations of conditions rather than the result of a single condition (‘conjunctural causation’)
-
Different possible configurations can lead to the same observed effects or outcomes: this is what QCA refers to as ‘equifinality’.
-
Causality is understood asymmetrically: if in a given case a certain condition is relevant for the outcome, its absence does not necessarily entail the absence of the outcome.
QCA belongs to the family of set theoretic methods. A case may be part of one or more sets. Sets articulate characteristics that certain cases may have in common. Building on our example, an employee attending an in-company training may be part of the set of cases of ‘employees with autonomy in their work and decision making’ and/or of the set of ’employees who received support from their supervisors in following the training’. Through identifying the extent to which a case is part of certain set and systematically comparing this with other cases with variation in the occurrence of a certain outcome (i.e. training transfer effectiveness), one can find out which (combinations of) factors are necessary and/or sufficient for this outcome:
-
A (combination) of condition(s) found as necessary implies that it will always be present/absent whenever the outcome is present/absent. Or to put it in terms of set theory, X is a necessary condition for Y, if Y is a subset of X (X ← Y). For example, if we would find that all in-company training leading to training transfer effectiveness were lectured by instructors with a lot of teaching experience, the latter can be qualified as a necessary condition.
-
In order for a condition (or a combination of conditions, i.e., configuration) to be sufficient, the outcome should appear whenever the condition is present. In set theory, a condition (X) is classified as sufficient if it constitutes a subset of the outcome (X → Y). For instance, a training attended by an employee with high autonomy in their work, and who is strongly motivated can constitute a sufficient path to training transfer effectiveness.
Cases can take different forms in QCA. In an evaluation setting, cases are commonly contexts in which an intervention has been applied. In the example mentioned earlier, cases relate to employees who attended a subsidised training. Cases can also be organisations or firms, or be situated at the macro-level (i.e. countries).
How then to compare such cases systematically? One can hereto resort to different QCA techniques. In the crisp set QCA (csQCA), the original version of QCA, conditions and outcome need to be translated in binary terms, 1 or 0. This is called calibration. Conditions or outcomes assigned a score of 1 should be read as present (or high, or large), while those with a score of 0 are regarded as absent (or low, or small). The binary scores express qualitative differences in kind. In the fuzzy set variant of QCA (fsQCA), cases can have partial membership in a set and any score between 0 and 1, which takes account of the fact that many social phenomena are dichotomous ‘in principle’ but that empirical manifestations of these phenomena in practice often differ in degree (Schneider, and Wagemann 2012, 14).
Irrespective the technique that is used, the QCA research cycle runs through similar stages:
First, with the data being calibrated, a data matrix can be constructed which basically presents the empirically observed data as a list of configurations.
Second, the calibrated data matrix can, in a subsequent stage, be transformed into a so-called truth table, which lists all possible configurations leading to a particular outcome. As a single configuration possibly corresponds with various empirical cases, the truth table thus summarises the empirical data table. The total number of theoretically possible configurations in the truth table is determined by the number of conditions included in the research. Researchers should strive for a good balance between the number of cases and conditions. The configurations not covered by empirical observations can be considered logical remainders, that is, they are logically possible, yet not observed. QCA provides the interesting opportunity to include plausible assumptions about the outcome of (a selection of) logical remainders for drawing more parsimonious inference (Schneider, and Wagemann 2012).
Third, the truth table paves the way to proceed to the QCA analytical moment, coined as Boolean minimisation. In this process, the researcher can rely on different software packages. The minimisation is built upon the assumption that if two combinations differ on only one condition, but show the same outcome, this particular condition is redundant. Thus, it can be eliminated to obtain a simpler representation of the case (or group of cases). Applying this rule iteratively on all possible pairs of combinations until no further simplification is possible results in a series of sufficient paths to the outcome. The type of findings (i.e. solution formulas) typically resulting from the QCA analysis will be expressions about ‘the (combination of) conditions that are necessary and/or sufficient for the occurrence or non-occurrence of a particular outcome’.
Fourth, and most crucially, a QCA study does not stop after the application of the software. It is essential that the researcher spells out the causal link in a narrative fashion (Schneider, and Wagemann 2010), by returning to the individual cases and by relating the findings with broader theoretical and conceptual knowledge. Common to the method is its iterative nature: researchers can go back and forth between preliminary data analysis and the dataset or the theory of change. This process is also a useful vehicle to get to know the cases in more depth.
II. How is this method useful for policy evaluation?
QCA can be used for explanatory purposes which enables one to test program theories in a systematic way, or for exploratory purposes to develop theories from case-based knowledge. As the outcome (effects) should be known before the evaluation, the method can in principle only be applied for ex post and in itinere evaluations, in which an intervention has led to variation in success.
By its focus, QCA is primarily suitable for learning rather than accountability-oriented evaluations. In particular, it is often said that it can contribute to both double loop and triple loop learning: it not only sheds light on the conditions under which policy interventions work; yet it also provides potential to actively involve stakeholders in the process of selecting outcomes, conditions, and in calibrating these. As a result, stakeholders and commissioners can get a better understanding of what ‘successful change’ means in the context of the intervention, and of what makes a difference.
III. An example of the use of this method in training policy
We already hinted at an evaluation about the effectiveness of soft skills training (such as leadership skills). This evaluation was conducted in Flemish (Belgian) firms, commissioned by the Flemish European Social Fund (ESF) agency, which also subsidised the training. Although previous counterfactual research demonstrated the positive impact of training subsidies, it also revealed that there is not always transfer of what is learned to the working environment. This observation constituted the main rationale for the evaluation and triggered the commissioner to switch focus from ‘whether the subsidised training works’ to the conditions under which training programmes work. The study included 50 cases, of which 15 successful cases in which social skills were transferred and 35 failed cases where training transfer effectiveness was not achieved at the time of evaluation.
Based on relevant educational literature, 8 conditions were identified with potential explanatory power and included in our QCA model: (1) peer support; (2) supervisor support; (3) sense of urgency; (4) relapse prevention and goal setting; and the following contextual conditions: (5) identical elements, (6) training program as active learning method; (7) autonomy, and (8) balanced workload. Of these conditions, no single condition proved necessary for successful training transfer. However, we identified several pathways consisting of combinations of conditions that were sufficient to success: whenever these pathways were present, the training content was successfully retained and applied to the workplace. Table 1 below visualises the eight pathways found.
The QCA analysis proved useful to know which conditions to monitor in future subsidised programmes. The evaluation was a component of a multimethod evaluation: it was followed by Process Tracing (see separate chapter on process tracing) which focused on a selection of cases to identify the mechanisms through which training programmes make a difference in the working environment. The QCA analysis also helped systematically identify the cases for which further in-depth within-case analysis was most relevant.
Table 1: Pathways to successful training transfer
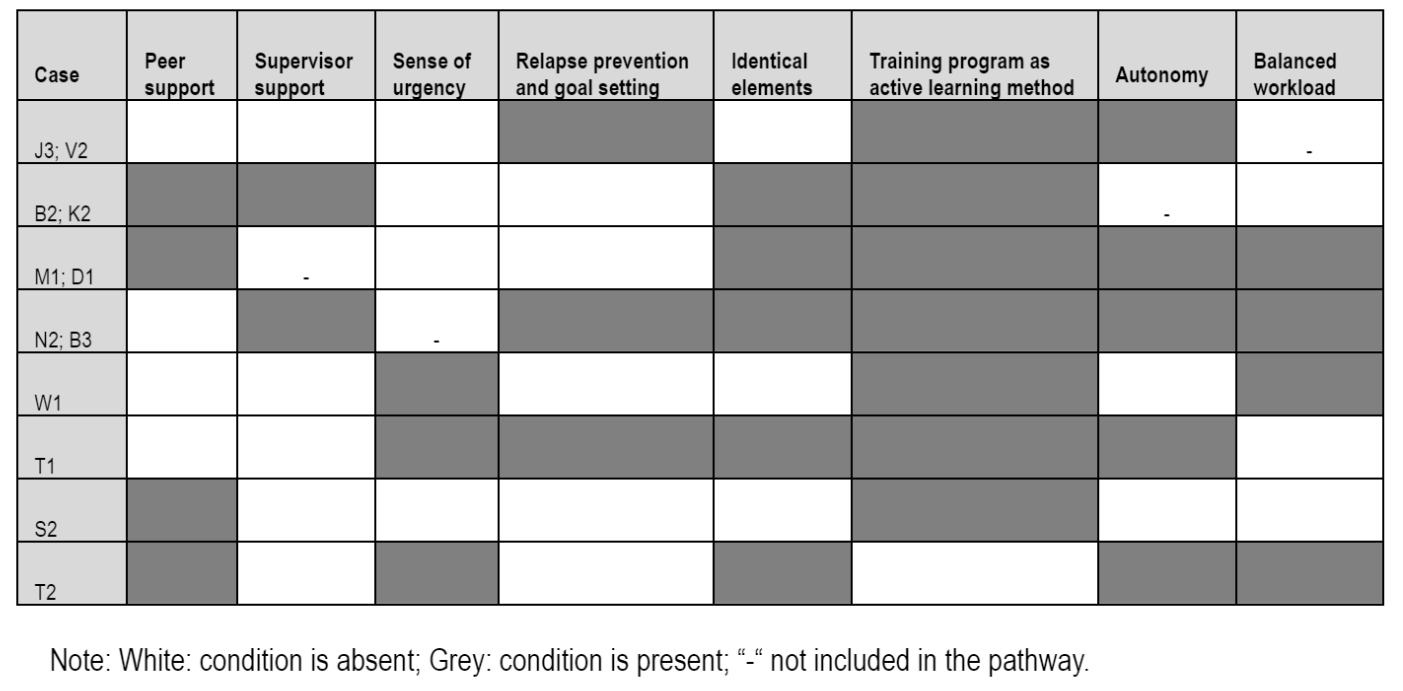
IV. What are the criteria for judging the quality of the mobilisation of this method?
Several checklists circulate with overviews of what QCA good practice involves (Schneider, and Wagemann 2010; Befani 2016: 183-185), and it would exceed the scope of this methodological sheet to elaborate on all quality criteria. It is imperative that QCA as a data analysis technique is applied consistently with the ‘spirit’ of QCA as a research approach, which implies that QCA should not be reduced to a mechanistic ‘push button process’. Besides, evaluators should be transparent about all choices made in the research process, and ideally resort to robustness tests for all decisions made. The latter is particularly important, given the strong case-sensitivity of the method.
The QCA analysis will generate different parameters of fit which help evaluating the analyses of necessity and sufficiency. Simply put, consistency describes the extent to which an empirical relationship between a (combination of) condition(s) and the outcome approximates set-theoretic necessity and/or sufficiency. Coverage describes the empirical importance or the relevance of a (combination) of condition(s). For necessary conditions, consistency is typically set very high, at 0.9; whereas for sufficient conditions, lower consistency values (e.g. 0.75) are relatively common. Coverage values should usually be 0.60 or higher. Importantly, however, the thresholds for what is deemed ‘good’ can vary with the research design and aim of the research (Schneider, and Wagemann 2010).
V. What are the strengths and limitations of this method compared to others?
QCA has the unique advantage to account for causal complexity, while also allowing for modest generalisation by the systematic identification of cross-case patterns. The rigorous procedures it relies on also makes the findings perfectly replicable. An additional advantage is that it does not need a large number of cases to be applied.
Strictly speaking, however, QCA will only unravel ‘associations’ between a condition and an outcome. The actual causal interpretation is up to the evaluators themselves. A similar limitation applies to the time element. Although various ways of including ‘time’ in a QCA analysis are being worked on (see Verweij, and Vis 2021), the type of findings is static in nature rather than dynamic.
Just because of these reasons, it is advisable to combine QCA with other within-case methods that have the ability to open the causal black box. In particular, the combination of QCA and Process Tracing is increasingly used for this purpose. The evaluation referred to in this methodological sheet is an example of this.
Some bibliographical references to go further
Befani, Barbara. 2016. “Pathways to change: Evaluating development interventions with Qualitative Comparative Analysis (QCA).” Sztokholm: Expertgruppen för biståndsanalys (the Expert Group for Aid Studies). Pobrane: http://eba.se/en/pathways-to-change-evaluating-development-interventions-with-qualitative-comparative-analysis-qca
Rihoux, Benoît. and Ragin, Charles C.. 2008. Configurational comparative methods: Qualitative comparative analysis (QCA) and related techniques. Sage Publications.
Schneider, Carsten. and Wagemann, Claudius. 2010. “Standards of good practice in qualitative comparative analysis (QCA) and fuzzy-sets.” Comparative sociology, 9, no.3: 397-418.
Schneider, Carsten. and Wagemann, Claudius. 2012. Set-theoretic methods for the social sciences: A guide to qualitative comparative analysis. Cambridge University Press.
Thomann, Eva. and Maggetti, Martino. 2020. “Designing research with qualitative comparative analysis (QCA): Approaches, challenges, and tools.” Sociological Methods & Research, 49, no.2: 356-386.
Verweij, Stefan. and Vis, Barbara. 2021. “Three strategies to track configurations over time with Qualitative Comparative Analysis.” European Political Science Review, 13, no.1: 95-111.
An excellent source is also www.compasss.org, which includes an extensive bibliography, an overview of software, tutorials and guidelines on QCA.